This article has been reviewed according to Science X's editorial process and policies. Editors have highlighted the following attributes while ensuring the content's credibility:
fact-checked
peer-reviewed publication
trusted source
proofread
How valuable are online product recommendations to consumers?
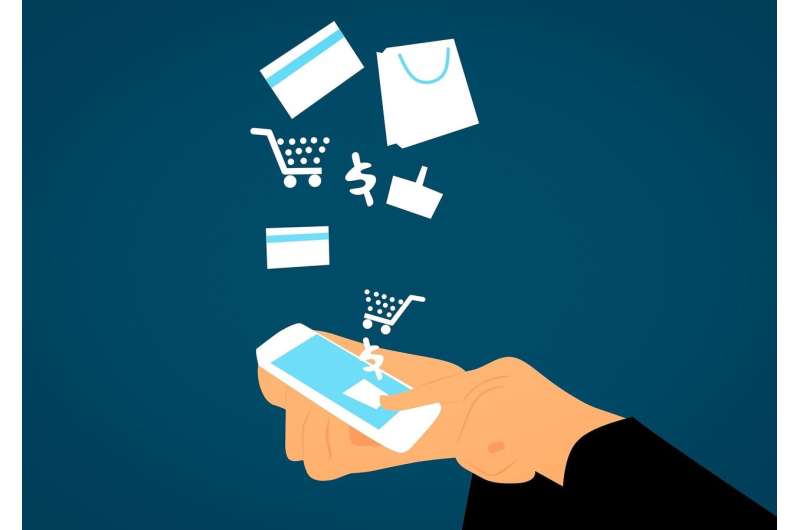
In today's online world, third parties collect and store your browsing data at staggering rates. Third parties benefit greatly from this information, but do you get fair returns for sharing your data online?
New research from the University of Florida Matherly Professor Anuj Kumar and Santa Clara University's Xiang (Shawn) Wan has proposed a novel method to measure the value consumers get from product recommendation systems (RS), one of the most prominent online tools that use consumer data.
While numerous studies have established that RS benefits retailers through increased sales, its benefits to consumers are unclear.
Presumably, RS algorithms are designed to help consumers find products that provide them with higher value among numerous choices. Consumers may value a product because of its higher quality (such as better material and craftsmanship), lower price (for a given quality), or better match with their tastes (such as their color choice). However, how much a consumer values a product is only known to her and not observed by others.
For example, imagine you are searching for a women's top on Macy's website. After searching, you purchased a red V-neck fitted top that you found organically (without RS) and a maroon collared top with a loose fit that you found with RS. The relative values of the two tops are only known to you. Maybe you liked the maroon color more than red or the loose fit more than the tight fit. Or you attached a higher value (higher quality) to the brand/designer of the maroon top than the red top. However, these facts were not apparent to the online retailer.
The difference in desirability (value) of products you found with and without RS is the true value of the RS. However, since no one observes the value you derive from these products, measuring how valuable RS is to consumers is a challenge.
Kumar and Wan, along with Xitong Li at HEC Paris, propose a novel method of measuring the value of RS to consumers from the similarity scores between products (called affinity scores) computed by RS algorithms in a forthcoming research article in Management Science, titled "How do recommendations help consumers search products? Evidence from a field experiment."
"On average, consumers purchase recommendations with higher affinity scores with the product they explore. Thus, affinity scores of recommended products measure their relative value to consumers," Kumar explained.
Kumar and his co-authors exploit this idea in a large-scale randomized study on a U.S. fashion apparel and home goods retailer's website, exploring millions of consumer products over nine weeks. They collected unique data on the affinity scores computed by an item-based Collaborative Filtering algorithm to develop measures of a product's net value and taste fit to consumers.
In this research, Kumar and his co-authors show that consumers are more likely to purchase under RS because it helps them find higher-value products—which are either lower-priced, match their tastes better, or both. They found that the benefit of RS was higher for product categories with high price dispersions and consumer taste heterogeneities. In addition, the research team found that consumers preferred to use RS over other search tools on the website in the data, perhaps because they found RS valuable.
Kumar affirms that policymakers can use their method to ascertain how consumers and retailers share the surplus created by the recommendations systems. The proposed method can compare existing RS in their abilities to help consumers discover high-value products and offer guidance on design improvements.
The research, "How do recommendations help consumers search products? Evidence from a field experiment," is forthcoming in Management Science.
Journal information: Management Science
Provided by University of Florida