This article has been reviewed according to Science X's editorial process and policies. Editors have highlighted the following attributes while ensuring the content's credibility:
fact-checked
trusted source
proofread
A machine learning model for identifying new compounds to fight against global warming
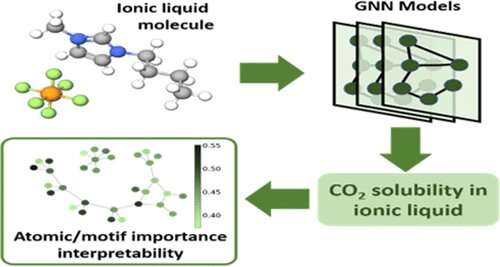
Among all greenhouse gases, carbon dioxide is the highest contributor to global warming. If we do not take action by 2100, according to the Intergovernmental Panel on Climate Change, the average temperature of our world will increase by about 34 degrees Fahrenheit. Finding effective ways to capture and store CO2 has been a challenge for researchers and industries focused on combating global warming, and Amir Barati Farimani has been working to change that.
"Machine learning models bear the promise for discovering new chemical compounds or materials to fight against global warming," explains Barati Farimani, an assistant professor of mechanical engineering at Carnegie Mellon University. "Machine learning models can achieve accurate and efficient virtual screening of CO2 storage candidates and may even generate preferable compounds that never existed before."
Barati Farimani has made a breakthrough using machine learning to identify ionic liquid molecules. Ionic liquids (ILs) are families of molten salt that remain in a liquid state at room temperature, have high chemical stability and high CO2 solubility, making them ideal candidates for CO2 storage. The combination of ions largely determines the properties of ILs. However, such combinatorial possibilities of cations and anions make it extremely challenging to exhaust the design space of ILs for efficient CO2 storage through conventional experiments.
Machine learning is often used in drug discovery to create so-called molecular fingerprints alongside graph neural networks (GNNs) that treat molecules as graphs and use a matrix to identify molecular bonds and related properties. For the first time, Barati Farimani has developed both fingerprint-based ML models and GNNs that are able to predict the CO2 absorption in ionic liquids.
"Our GNN method achieves superior accuracy in predicting the CO2 solubility in ion liquids," states Barati Farimani. "Unlike previous ML methods that rely on handcrafted features, GNN directly learns the features from molecular graphs."
Understanding how machine learning models make decisions is just as important as the molecular properties they identify. This explanation provides researchers with extra insight into how the structure of the molecule affects the property of ionic liquids from a data-driven perspective. For example, Barati Farmimani's team found that molecular fragments that physically interact with CO2 are less important than those that have a chemical interaction. Additionally, those with less hydrogen connected to nitrogen could be more favorable in formalizing a stable chemical interaction with CO2.
These findings, published in ACS Sustainable Chemistry & Engineering, will enable researchers to advise on the design of novel and efficient ionic liquids for CO2 storage in the future.
More information: Yue Jian et al, Predicting CO2 Absorption in Ionic Liquids with Molecular Descriptors and Explainable Graph Neural Networks, ACS Sustainable Chemistry & Engineering (2022). DOI: 10.1021/acssuschemeng.2c05985