This article has been reviewed according to Science X's editorial process and policies. Editors have highlighted the following attributes while ensuring the content's credibility:
fact-checked
trusted source
proofread
Deep learning for quantum sensing
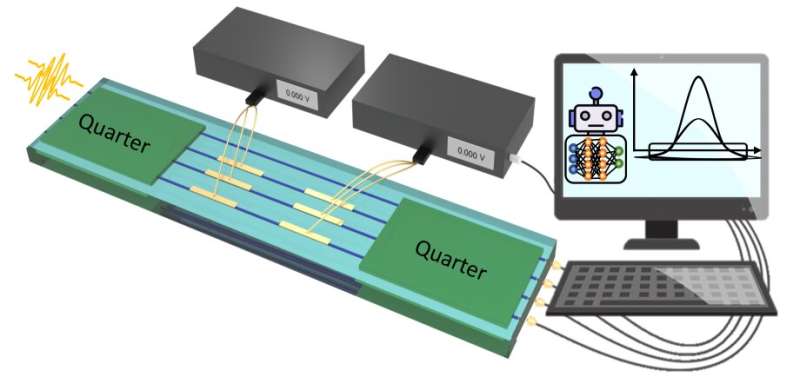
Quantum sensing represents one of the most promising applications of quantum technologies, with the aim of using quantum resources to improve measurement sensitivity. In particular, sensing of optical phases is one of the most investigated problems, considered key to developing mass-produced technological devices.
Optimal usage of quantum sensors requires regular characterization and calibration. In general, such calibration is an extremely complex and resource-intensive task—especially when considering systems for estimating multiple parameters, due to the sheer volume of required measurements as well as the computational time needed to analyze those measurements. Machine-learning algorithms present a powerful tool to address that complexity. The discovery of suitable protocols for algorithm usage is vital for the development of sensors for precise quantum-enhanced measurements.
A particular type of machine-learning algorithm known as "reinforcement learning" (RL) relies on an intelligent agent guided by rewards: Depending on the rewards it receives, it learns to perform the right actions to achieve the desired optimization. The first experimental realizations using RL algorithms for the optimization of quantum problems have been reported only very recently. Most of them still rely on prior knowledge of the model describing the system. What is desirable is instead a completely model-free approach, which is possible when the agent's reward does not depend on the explicit system model.
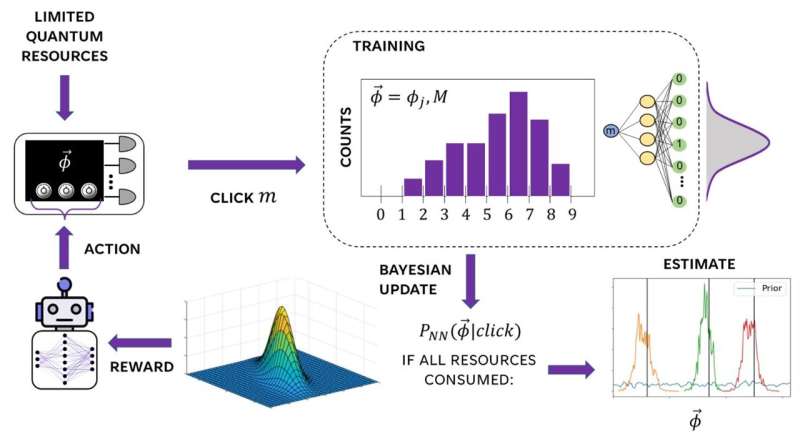
As reported in Advanced Photonics, a team of researchers from the Physics Department of Sapienza University of Rome and the Institute for Photonics and Nanotechnologies (IFN-CRN) has recently developed a model-free approach that widens the range of possible applications to that of adaptive multiphase estimation. The team demonstrates the effectiveness of their model-free approach in a highly reconfigurable integrated photonic platform. They experimentally employ the RL algorithm to optimize estimation of multiple parameters and combine it with a deep neural network that updates after each measurement the Bayesian posterior probability distribution.
The protocol handles the quantum multiparameter sensor in a completely black-box manner, since at any step the system functioning model is not required. Importantly, the team proves the enhanced performance obtained with their protocol on experimental data in a resource-limited regime and compares it to that of non-adaptive strategies, achieving significantly better estimations.
According to corresponding author Fabio Sciarrino, head of the Quantum Lab, "The protocol developed by our team provides a significant step toward fully artificial-intelligence-based quantum sensors."
More information: Valeria Cimini et al, Deep reinforcement learning for quantum multiparameter estimation, Advanced Photonics (2023). DOI: 10.1117/1.AP.5.1.016005
Provided by SPIE